import numpy as np
import matplotlib as mpl
from mpl_toolkits.mplot3d import Axes3D
from matplotlib import cm
import time
from scipy.optimize import leastsq
from scipy import stats
import scipy.optimize as opt
import matplotlib.pyplot as plt
from scipy.stats import norm, poisson
from scipy.interpolate import BarycentricInterpolator
from scipy.interpolate import CubicSpline
import scipy as sp
import math
def residual(t, x, y):
return y - (t[0] * x ** 2 + t[1] * x + t[2])
def residual2(t, x, y):
print(t[0], t[1])
return y - (t[0]*np.sin(t[1]*x) + t[2])
def f(x):
y = np.ones_like(x)
i = x > 0
y[i] = np.power(x[i], x[i])
i = x < 0
y[i] = np.power(-x[i], -x[i])
return y
a = np.arange(0, 60, 10).reshape((-1, 1)) + np.arange(6)
print(a)
[[ 0 1 2 3 4 5]
[10 11 12 13 14 15]
[20 21 22 23 24 25]
[30 31 32 33 34 35]
[40 41 42 43 44 45]
[50 51 52 53 54 55]]
- 标准Python的列表(list)中,元素本质是对象。
- 如:L = [1, 2, 3],需要3个指针和三个整数对象,对于数值运算比较浪费内存和CPU。
- 因此,Numpy提供了ndarray(N-dimensional array object)对象:存储单一数据类型的多维数组
1.使用array创建
L = [1, 2, 3, 4, 5, 6]
print('L = ', L)
a = np.array(L)
print('a = ', a)
print(type(a),type(L))
L = [1, 2, 3, 4, 5, 6]
a = [1 2 3 4 5 6]
b = np.array([[1, 2, 3, 4], [5, 6, 7, 8], [9, 10, 11, 12]])
print(b)
[[ 1 2 3 4]
[ 5 6 7 8]
[ 9 10 11 12]]
print(a.shape)
print(b.shape)
[[ 1 2 3]
[ 4 5 6]
[ 7 8 9]
[10 11 12]]
b.shape = 2, -1
print(b)
print(b.shape)
[[ 1 2 3 4 5 6]
[ 7 8 9 10 11 12]]
(2, 6)
b.shape = 3, 4
print(b)
c = b.reshape((4, -1))
print('b = \n', b)
print('c = \n', c)
[[ 1 2 3 4]
[ 5 6 7 8]
[ 9 10 11 12]]
b =
[[ 1 2 3 4]
[ 5 6 7 8]
[ 9 10 11 12]]
c =
[[ 1 2 3]
[ 4 5 6]
[ 7 8 9]
[10 11 12]]
c[1][2] = 600
print('b = \n', b)
print('c = \n', c)
b =
[[ 1 2 3 4]
[ 5 600 7 8]
[ 9 10 11 12]]
c =
[[ 1 2 3]
[ 4 5 600]
[ 7 8 9]
[ 10 11 12]]
print(a.dtype)
print(b.dtype)
d = np.array([[1, 2, 3, 4], [5, 6, 7, 8], [9, 10, 11, 12]], dtype=np.float)
f = np.array([[1, 2, 3, 4], [5, 6, 7, 8], [9, 10, 11, 12]], dtype=np.complex)
print(d)
print(f)
[[ 1. 2. 3. 4.]
[ 5. 6. 7. 8.]
[ 9. 10. 11. 12.]]
[[ 1.+0.j 2.+0.j 3.+0.j 4.+0.j]
[ 5.+0.j 6.+0.j 7.+0.j 8.+0.j]
[ 9.+0.j 10.+0.j 11.+0.j 12.+0.j]]
f = d.astype(np.int)
print(f)
[[ 1 2 3 4]
[ 5 6 7 8]
[ 9 10 11 12]]
2.使用函数创建
a = np.arange(1,20,3)
print(a)
print(type(a),a.dtype)
[ 1 4 7 10 13 16 19]
int32
b = np.linspace(1, 10, 10)
print('b = ', b)
b = [ 1. 2. 3. 4. 5. 6. 7. 8. 9. 10.]
c = np.linspace(1, 10, 10, endpoint=False)
print('c = ', c)
c = [ 1. 1.9 2.8 3.7 4.6 5.5 6.4 7.3 8.2 9.1]
d = np.logspace(1, 4, 4, endpoint=True,base=2)
print('d = ', d)
np.set_printoptions(suppress=True, linewidth=150)
f = np.logspace(0, 10 ,11, endpoint=True,base=2)
print('f = ', f)
f = [ 1. 2. 4. 8. 16. 32. 64. 128. 256. 512. 1024.]
s = 'abcdABCDEF'
g = np.fromstring(s, dtype=np.int8)
print(g)
[ 97 98 99 100 65 66 67 68 69 70]
3.存取
a = np.arange(10)
print(a)
a[1:4] = 10, 20, 30
print(a)
[ 0 10 20 30 4 5 6 7 8 9]
b = a[2:5]
print(b)
b[0] = 100
print(a)
[100 30 4]
[ 0 10 100 30 4 5 6 7 8 9]
3.2 整数/布尔数组存取
a = np.logspace(0, 9, 10, base=2)
print(a)
i = np.arange(0, 10, 2)
print(i)
b = a[i]
print(b)
b[2] = 1.6
print(b)
print(a)
[ 1. 2. 4. 8. 16. 32. 64. 128. 256. 512.]
[0 2 4 6 8]
[ 1. 4. 16. 64. 256.]
[ 1. 4. 1.6 64. 256. ]
[ 1. 2. 4. 8. 16. 32. 64. 128. 256. 512.]
np.set_printoptions(linewidth=200)
a = np.random.rand(10)
print(a)
[ 0.50849212 0.89850527 0.25803128 0.09660402 0.96200096 0.48726367 0.05403987 0.50161325 0.96409502 0.82349272]
[ True True False False True False False True True True]
[ 0.50849212 0.89850527 0.96200096 0.50161325 0.96409502 0.82349272]
a[a > 0.5] = 0.5
print(a)
[ 0.5 0.5 0.25803128 0.09660402 0.5 0.48726367 0.05403987 0.5 0.5 0.5 ]
3.3 二维数组的切片
a = np.arange(0,60,10)
print('a = ', a)
b= a.reshape((-1, 1))
print('b = ', b)
b = [[ 0]
[10]
[20]
[30]
[40]
[50]]
c = np.arange(6)
print('c = ', c)
f = b + c
print(f)
c = [0 1 2 3 4 5]
[[ 0 1 2 3 4 5]
[10 11 12 13 14 15]
[20 21 22 23 24 25]
[30 31 32 33 34 35]
[40 41 42 43 44 45]
[50 51 52 53 54 55]]
a = np.arange(0, 60, 10).reshape((-1, 1)) + np.arange(6)
print(a)
[[ 0 1 2 3 4 5]
[10 11 12 13 14 15]
[20 21 22 23 24 25]
[30 31 32 33 34 35]
[40 41 42 43 44 45]
[50 51 52 53 54 55]]
print(a[[0, 1, 2], [2, 3, 4]])
print(a[4, [2, 3, 4]])
print(a[4:, [2, 3, 4]])
[ 2 13 24]
[42 43 44]
[[42 43 44]
[52 53 54]]
i = np.array([True, False, True, False, False, True])
print(a[i])
print(a[i, 3])
[[ 0 1 2 3 4 5]
[20 21 22 23 24 25]
[50 51 52 53 54 55]]
[ 3 23 53]
4.1 numpy与Python数学库的时间比较
for j in np.logspace(0, 7, 8):
x = np.linspace(0, 10, j)
start = time.clock()
y = np.sin(x)
t1 = time.clock() - start
x = x.tolist()
start = time.clock()
for i, t in enumerate(x):
x[i] = math.sin(t)
t2 = time.clock() - start
print(j, ": ", t1, t2, t2/t1)
C:\Program Files\Anaconda3\lib\site-packages\ipykernel\__main__.py:2: DeprecationWarning: object of type cannot be safely interpreted as an integer.
from ipykernel import kernelapp as app
10000000.0 : 0.17834738633189676 4.184879334368658 23.464764022842356
4.2 元素去重
a = np.array((1, 2, 3, 4, 5, 5, 7, 3, 2, 2, 8, 8))
print('原始数组:', a)
原始数组: [1 2 3 4 5 5 7 3 2 2 8 8]
b = np.unique(a)
print('去重后:', b)
c = np.array(((1, 2), (3, 4), (5, 6), (1, 3), (3, 4), (7, 6)))
print('二维数组:\n', c)
print('去重后:', np.unique(c))
print('去重方案2:\n', np.array(list(set([tuple(t) for t in c]))))
二维数组:
[[1 2]
[3 4]
[5 6]
[1 3]
[3 4]
[7 6]]
去重后: [1 2 3 4 5 6 7]
去重方案2:
[[1 2]
[7 6]
[1 3]
[3 4]
[5 6]]
4.3 stack and axis 堆和轴
a = np.arange(1, 7).reshape((2, 3))
b = np.arange(11, 17).reshape((2, 3))
c = np.arange(21, 27).reshape((2, 3))
d = np.arange(31, 37).reshape((2, 3))
print('a = \n', a)
print('b = \n', b)
print('c = \n', c)
print('d = \n', d)
a =
[[1 2 3]
[4 5 6]]
b =
[[11 12 13]
[14 15 16]]
c =
[[21 22 23]
[24 25 26]]
d =
[[31 32 33]
[34 35 36]]
s = np.stack((a, b, c, d), axis=0)
print('axis = 0 ', s.shape, '\n', s)
axis = 0 (4, 2, 3)
[[[ 1 2 3]
[ 4 5 6]]
[[11 12 13]
[14 15 16]]
[[21 22 23]
[24 25 26]]
[[31 32 33]
[34 35 36]]]
s = np.stack((a, b, c, d), axis=1)
print('axis = 1 ', s.shape, '\n', s)
axis = 1 (2, 4, 3)
[[[ 1 2 3]
[11 12 13]
[21 22 23]
[31 32 33]]
[[ 4 5 6]
[14 15 16]
[24 25 26]
[34 35 36]]]
s = np.stack((a, b, c, d), axis=2)
print('axis = 2 ', s.shape, '\n', s)
axis = 2 (2, 3, 4)
[[[ 1 11 21 31]
[ 2 12 22 32]
[ 3 13 23 33]]
[[ 4 14 24 34]
[ 5 15 25 35]
[ 6 16 26 36]]]
a = np.arange(1, 10).reshape(3,3)
print('a = ', a)
b = a + 10
print('b = ', b)
print(np.dot(a, b))
print(a * b)
a = [[1 2 3]
[4 5 6]
[7 8 9]]
b = [[11 12 13]
[14 15 16]
[17 18 19]]
[[ 90 96 102]
[216 231 246]
[342 366 390]]
[[ 11 24 39]
[ 56 75 96]
[119 144 171]]
a = np.arange(1, 10)
print(a)
b = np.arange(20, 25)
print(b)
print(np.concatenate((a, b)))
[1 2 3 4 5 6 7 8 9]
[20 21 22 23 24]
[ 1 2 3 4 5 6 7 8 9 20 21 22 23 24]
5.绘图
mpl.rcParams['font.sans-serif'] = [u'SimHei']
mpl.rcParams['axes.unicode_minus'] = False
mu = 0
sigma = 1
x = np.linspace(mu - 3 * sigma, mu + 3 * sigma, 51)
y = np.exp(-(x - mu) ** 2 / (2 * sigma ** 2)) / (math.sqrt(2 * math.pi) * sigma)
print(x.shape)
print('x = \n', x)
print(y.shape)
print('y = \n', y)
plt.figure(facecolor='w')
plt.plot(x, y, 'ro-', linewidth=2)
plt.xlabel('X', fontsize=15)
plt.ylabel('Y', fontsize=15)
plt.title(u'正态函数分布', fontsize=18)
plt.grid(True)
plt.show()
(51,)
x =
[-3. -2.88 -2.76 -2.64 -2.52 -2.4 -2.28 -2.16 -2.04 -1.92 -1.8 -1.68 -1.56 -1.44 -1.32 -1.2 -1.08 -0.96 -0.84 -0.72 -0.6 -0.48 -0.36 -0.24 -0.12 0. 0.12 0.24 0.36 0.48 0.6 0.72 0.84
0.96 1.08 1.2 1.32 1.44 1.56 1.68 1.8 1.92 2.04 2.16 2.28 2.4 2.52 2.64 2.76 2.88 3. ]
(51,)
y =
[ 0.00443185 0.00630673 0.00884645 0.01223153 0.0166701 0.02239453 0.02965458 0.03870686 0.04980009 0.06315656 0.07895016 0.09728227 0.1181573 0.14145997 0.16693704 0.19418605
0.2226535 0.25164434 0.28034381 0.30785126 0.3332246 0.35553253 0.37391061 0.38761662 0.39608021 0.39894228 0.39608021 0.38761662 0.37391061 0.35553253 0.3332246 0.30785126
0.28034381 0.25164434 0.2226535 0.19418605 0.16693704 0.14145997 0.1181573 0.09728227 0.07895016 0.06315656 0.04980009 0.03870686 0.02965458 0.02239453 0.0166701 0.01223153
0.00884645 0.00630673 0.00443185]
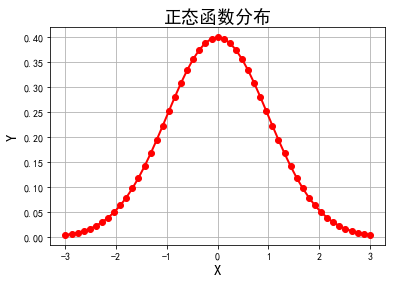
plt.figure(figsize=(10,8), facecolor='w')
x = np.linspace(start=-2, stop=3, num=1001, dtype=np.float)
y_logit = np.log(1 + np.exp(-x)) / math.log(2)
y_boost = np.exp(-x)
y_01 = x < 0
y_hinge = 1.0 - x
y_hinge[y_hinge < 0] = 0
plt.plot(x, y_logit, 'r-', label='Logistic Loss',linewidth=2)
plt.plot(x, y_01, 'g-', label='0/1 Loss', linewidth=2)
plt.plot(x, y_hinge, 'b-', label='Hinge Loss', linewidth=2)
plt.plot(x, y_boost, 'm--', label='Adaboost Loss', linewidth=2)
plt.grid()
plt.legend(loc='upper right')
plt.savefig('1.png')
plt.show()
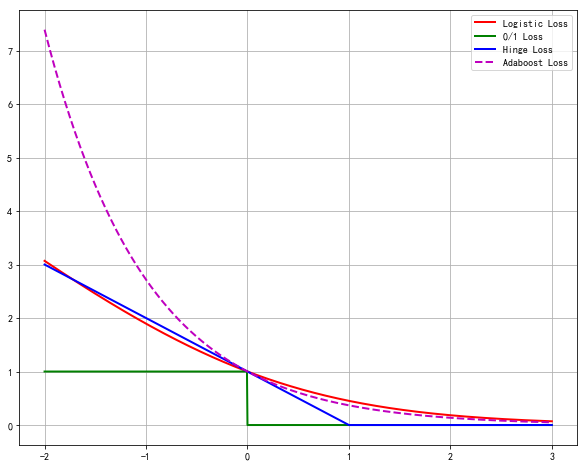
plt.figure(facecolor='w')
x = np.linspace(-1.3, 1.3, 101)
y = x
plt.plot(x, y, 'g-', label='x^x', linewidth=2)
plt.grid()
plt.legend(loc='upper left')
plt.show()
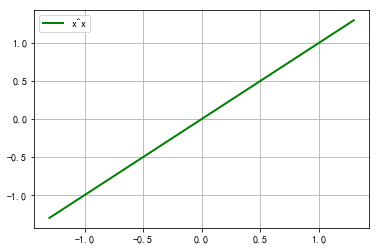
x = np.arange(1, 0, -0.01)
y = (-3 * x *np.log(x) + np.exp(-(40 * (x - 1 / np.e)) ** 4) / 25) / 2
plt.figure(figsize=(5, 7),facecolor='w')
plt.plot(y, x, 'r-', linewidth=2)
plt.grid(True)
plt.title(u'胸型线', fontsize=20)
plt.savefig('breast.png')
plt.show()

t = np.linspace(0, 2*np.pi, 100)
x = 16 * np.sin(t) ** 3
y = 13 * np.cos(t) - 5 * np.cos(2*t) - 2 * np.cos(3*t) - np.cos(4*t)
plt.plot(x, y, 'r-', linewidth=2)
plt.grid(True)
plt.show()
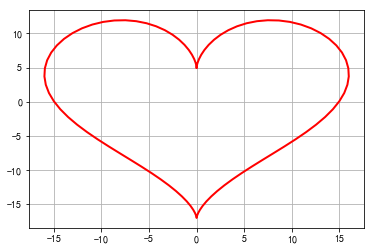
t = np.linspace(0, 50, num=1000)
x = t*np.sin(t) + np.cos(t)
y = np.sin(t) - t*np.cos(t)
plt.plot(x, y, 'r-', linewidth=2)
plt.grid()
plt.show()
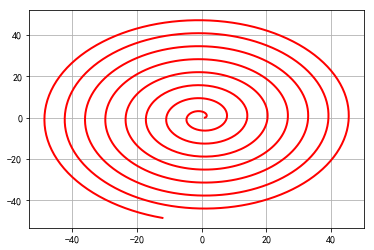
x = np.arange(0, 10, 0.1)
y = np.sin(x)
plt.bar(x, y, width=0.04, linewidth=0.2)
plt.plot(x, y, 'r--', linewidth=2)
plt.title(u'Sin曲线')
plt.xticks(rotation=0)
plt.xlabel('X')
plt.ylabel('Y')
plt.grid()
plt.show()
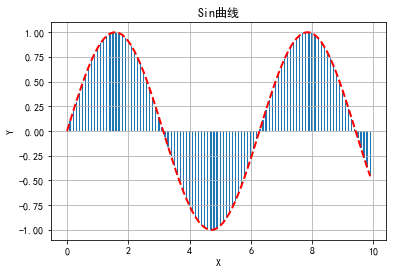
6. 概率分布
x = np.random.rand(10000)
t = np.arange(len(x))
plt.hist(x, 30, color='m', alpha=0.5, label=u'均匀分布')
plt.legend(loc='upper left')
plt.grid()
plt.show()
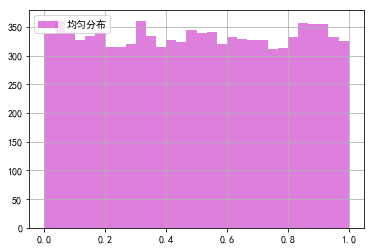
t = 1000
a = np.zeros(10000)
for i in range(t):
a += np.random.uniform(-5, 5, 10000)
a /= t
plt.hist(a, bins=30, color='g', alpha=0.5, normed=True, label=u'均匀分布叠加')
plt.legend(loc='upper left')
plt.grid()
plt.show()
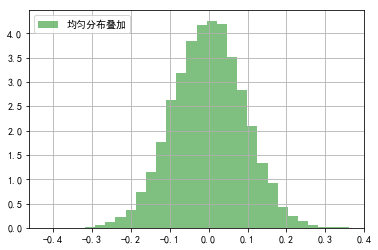
lamda = 7
p = stats.poisson(lamda)
y = p.rvs(size=1000)
mx = 30
r = (0, mx)
bins = r[1] - r[0]
plt.figure(figsize=(15, 8), facecolor='w')
plt.subplot(121)
plt.hist(y, bins=bins, range=r, color='g', alpha=0.8, normed=True)
t = np.arange(0, mx+1)
plt.plot(t, p.pmf(t), 'ro-', lw=2)
plt.grid(True)
N = 1000
M = 10000
plt.subplot(122)
a = np.zeros(M, dtype=np.float)
p = stats.poisson(lamda)
for i in np.arange(N):
a += p.rvs(size=M)
a /= N
plt.hist(a, bins=20, color='g', alpha=0.8, normed=True)
plt.grid(b=True)
plt.show()
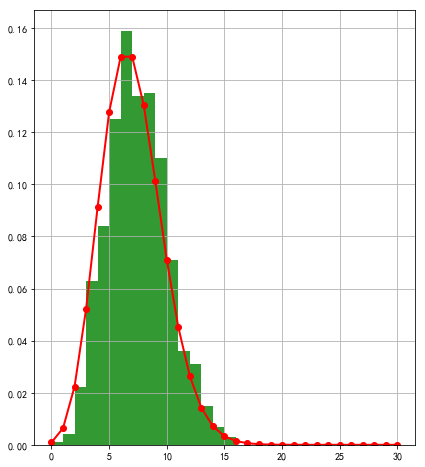
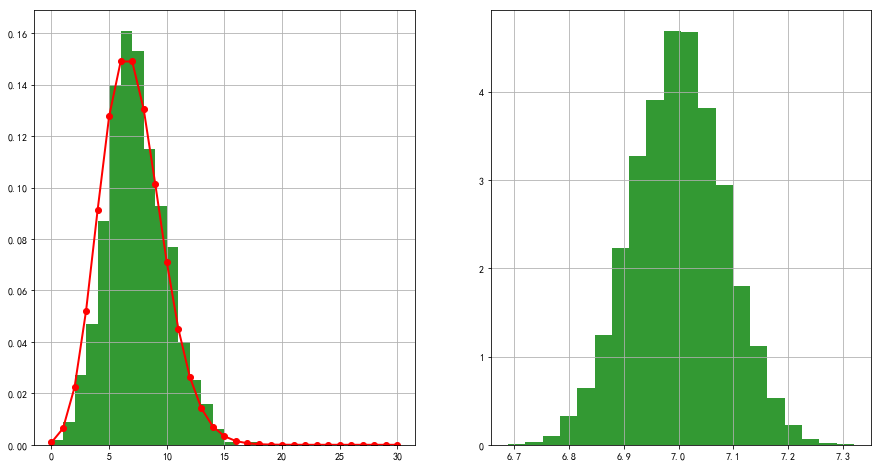
x = np.random.poisson(lam=5, size=10000)
print(x)
pillar = 15
a = plt.hist(x, bins=pillar, normed=True, range=[0, pillar], color='g', alpha=0.5)
plt.grid()
plt.show()
print(a)
print(a[0].sum())
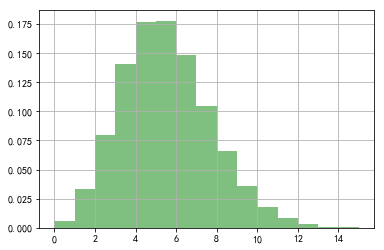
(array([ 0.0059, 0.0331, 0.0798, 0.1407, 0.1771, 0.1779, 0.1488, 0.1048, 0.066 , 0.0356, 0.0175, 0.0081, 0.0029, 0.0011, 0.0007]), array([ 0., 1., 2., 3., 4., 5., 6., 7., 8., 9., 10., 11., 12., 13., 14., 15.]), )
1.0
mu = 2
sigma = 3
data = mu + sigma * np.random.randn(1000)
h = plt.hist(data, 30, normed=1, color='#FFFFA0')
x = h[1]
y = norm.pdf(x, loc=mu, scale=sigma)
plt.plot(x, y, 'r-', x, y, 'ro', linewidth=2, markersize=4)
plt.grid()
plt.show()
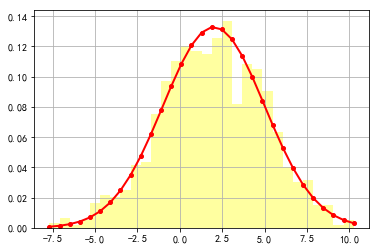
rv = poisson(5)
x1 = a[1]
y1 = rv.pmf(x1)
itp = BarycentricInterpolator(x1, y1)
x2 = np.linspace(x.min(), x.max(), 50)
y2 = itp(x2)
cs = sp.interpolate.CubicSpline(x1, y1)
plt.plot(x2, cs(x2), 'm--', linewidth=5, label='CubicSpine')
plt.plot(x2, y2, 'g-', linewidth=3, label='BarycentricInterpolator')
plt.plot(x1, y1, 'r-', linewidth=1, label='Actural Value')
plt.legend(loc='upper right')
plt.grid()
plt.show()

size = 1000
lamda = 5
p = np.random.poisson(lam=lamda, size=size)
plt.figure()
plt.hist(p, bins=range(3 * lamda), histtype='bar', align='left', color='r', rwidth=0.8, normed=True)
plt.grid(b=True, ls=':')
plt.title('Numpy.random.poisson', fontsize=13)
plt.figure()
r = stats.poisson(mu=lamda)
p = r.rvs(size=size)
plt.hist(p, bins=range(3 * lamda), color='r', align='left', rwidth=0.8, normed=True)
plt.grid(b=True, ls=':')
plt.title('scipy.stats.poisson', fontsize=13)
plt.show()

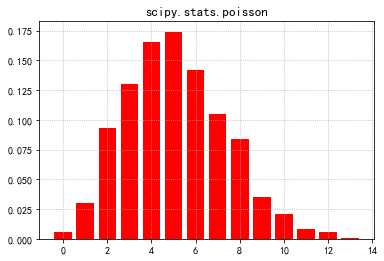
x, y = np.mgrid[-3:3:7j, -3:3:7j]
print(x)
print(y)
[[-3. -3. -3. -3. -3. -3. -3.]
[-2. -2. -2. -2. -2. -2. -2.]
[-1. -1. -1. -1. -1. -1. -1.]
[ 0. 0. 0. 0. 0. 0. 0.]
[ 1. 1. 1. 1. 1. 1. 1.]
[ 2. 2. 2. 2. 2. 2. 2.]
[ 3. 3. 3. 3. 3. 3. 3.]]
[[-3. -2. -1. 0. 1. 2. 3.]
[-3. -2. -1. 0. 1. 2. 3.]
[-3. -2. -1. 0. 1. 2. 3.]
[-3. -2. -1. 0. 1. 2. 3.]
[-3. -2. -1. 0. 1. 2. 3.]
[-3. -2. -1. 0. 1. 2. 3.]
[-3. -2. -1. 0. 1. 2. 3.]]
u = np.linspace(-3, 3, 101)
x, y = np.meshgrid(u, u)
print(x)
print(y)
z = x*y*np.exp(-(x**2 + y**2)/2) / math.sqrt(2*math.pi)
fig = plt.figure()
ax = fig.add_subplot(111, projection='3d')
ax.plot_surface(x, y, z, rstride=3, cstride=3, cmap=cm.Accent, linewidth=0.5)
plt.show()
[[-3. -2.94 -2.88 ..., 2.88 2.94 3. ]
[-3. -2.94 -2.88 ..., 2.88 2.94 3. ]
[-3. -2.94 -2.88 ..., 2.88 2.94 3. ]
...,
[-3. -2.94 -2.88 ..., 2.88 2.94 3. ]
[-3. -2.94 -2.88 ..., 2.88 2.94 3. ]
[-3. -2.94 -2.88 ..., 2.88 2.94 3. ]]
[[-3. -3. -3. ..., -3. -3. -3. ]
[-2.94 -2.94 -2.94 ..., -2.94 -2.94 -2.94]
[-2.88 -2.88 -2.88 ..., -2.88 -2.88 -2.88]
...,
[ 2.88 2.88 2.88 ..., 2.88 2.88 2.88]
[ 2.94 2.94 2.94 ..., 2.94 2.94 2.94]
[ 3. 3. 3. ..., 3. 3. 3. ]]
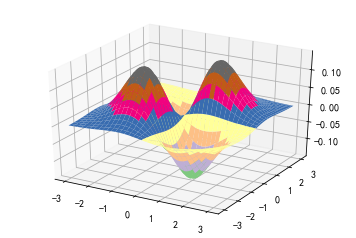
8.1 scipy
x = np.linspace(-2, 2, 50)
A, B, C = 2, 3, -1
y = (A * x ** 2 + B * x + C) + np.random.rand(len(x))*0.75
t = leastsq(residual, [0, 0, 0], args=(x, y))
theta = t[0]
print('真实值:', A, B, C)
print('预测值:', theta)
y_hat = theta[0] * x ** 2 + theta[1] * x + theta[2]
plt.plot(x, y, 'r-', linewidth=2, label=u'Actual')
plt.plot(x, y_hat, 'g-', linewidth=2, label=u'Predict')
plt.legend(loc='upper left')
plt.grid()
plt.show()
真实值: 2 3 -1
预测值: [ 2.05039352 2.98038567 -0.68426016]
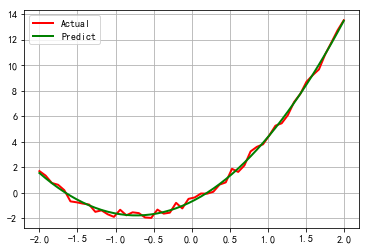
x = np.linspace(0, 5, 100)
a = 5
w = 1.5
phi = -2
y = a * np.sin(w*x) + phi + np.random.rand(len(x))*0.5
t = leastsq(residual2, [3, 5, 1], args=(x, y))
theta = t[0]
print('真实值:', a, w, phi)
print('预测值:', theta)
y_hat = theta[0] * np.sin(theta[1] * x) + theta[2]
plt.plot(x, y, 'r-', linewidth=2, label='Actual')
plt.plot(x, y_hat, 'g-', linewidth=2, label='Predict')
plt.legend(loc='lower left')
plt.grid()
plt.show()
3 5
3.0 5.0
3.0 5.0
3.0000000447 5.0
3.0 5.00000007451
3.0 5.0
-0.420957560799 5.01295154297
-0.420957554526 5.01295154297
-0.420957560799 5.01295161767
-0.420957560799 5.01295154297
-0.415107964139 4.88731071279
-0.415107964139 4.88731071279
-0.415107964139 4.88731071279
-0.417991597274 4.95413894637
-0.417991591045 4.95413894637
-0.417991597274 4.95413902019
-0.417991597274 4.95413894637
-0.429171220659 4.98637853424
-0.428676379387 4.97589257849
-0.428676373 4.97589257849
-0.428676379387 4.97589265264
-0.428676379387 4.97589257849
-0.428514583421 4.96500191779
-0.428514577035 4.96500191779
-0.428514583421 4.96500199177
-0.428514583421 4.96500191779
-0.42908509502 4.96683011246
-0.42908509502 4.96683011246
-0.429072886119 4.96657487607
-0.42902243432 4.96569756103
-0.429022427927 4.96569756103
-0.42902243432 4.96569763502
-0.42902243432 4.96569756103
-0.429038817978 4.9656407609
真实值: 5 1.5 -2
预测值: [-0.42902243 4.96569756 -1.29790843]
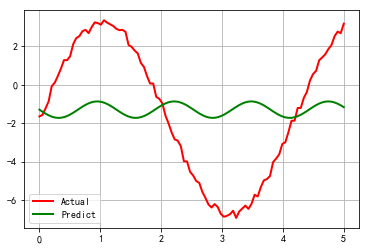
a = opt.fmin(f, 1)
b = opt.fmin_cg(f, 1)
c = opt.fmin_bfgs(f, 1)
print(a, 1/a, math.e)
print(b)
print(c)